What is zkML? Explanation & Use Cases
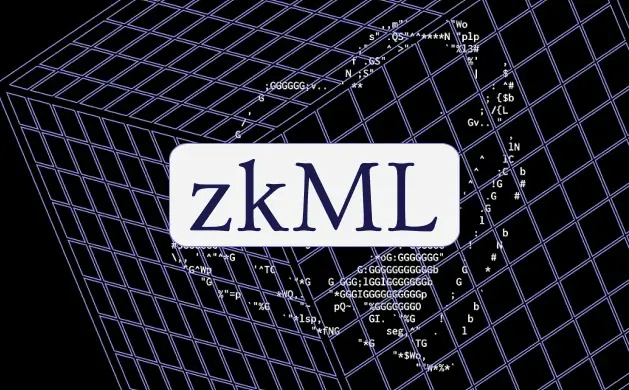
Summary: zkML established cryptographically enforceable trust in AI systems, with early adoption in DeFi and healthcare, but remains hindered by the computational strain of scaling to GPT-4-level models.
Investors are funneling capital into hardware accelerators and niche use cases, betting zkML will become critical infrastructure for industries where AI opacity risks systemic collapse.
What is Zero-Knowledge Machine Learning (zkML)?
Zero-Knowledge Machine Learning (zkML) merges zero-knowledge proofs (ZKPs), a cryptographic method for proving statements without revealing underlying data, with machine learning (ML) to enable privacy-preserving and verifiable computations.
zkML currently verifies smaller AI projects, like trading bots or image generators, using cryptographic proofs that confirm rule compliance. Scaling to large models (e.g., GPT-4o, o1, DALL-E 2, Claude 3.5, Grok-1) remains limited by extreme computational costs.
Additional applications might range from fraud detection to private biometric authentication. By using zero-knowledge proofs, zkML ensures privacy and computational integrity, making it a needed advancement for sensitive fields like finance (traditional/decentralized) and healthcare.
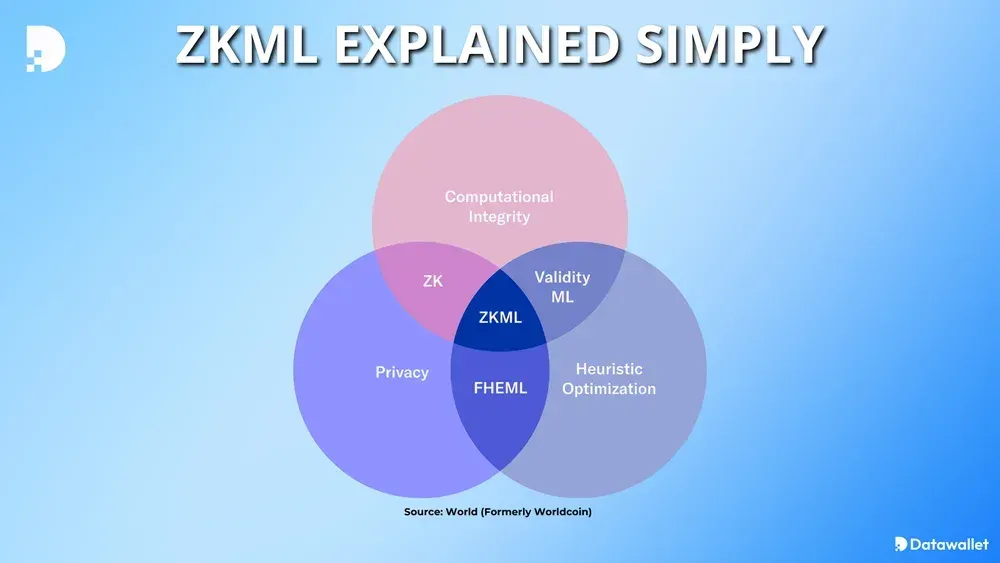
How Does zkML Work?
zkML enables a prover to confirm the correctness of an AI model's inference or training process without revealing sensitive details such as input data, model weights, or other private information.
The zkML verification process involves these key steps:
- Cryptographic Conversion: ML operations (inference, training) are translated into arithmetic circuits or constraint systems, transforming neural network layers into mathematical relations.
- Proof Generation: ZK-proof systems (zk-SNARKs, zk-STARKs) generate succinct cryptographic proofs attesting to the correctness of ML computations, hashing non-linear activations, matrix multiplications, and data flows into algebraic statements.
- Verification: Third parties validate proofs against public parameters (e.g., model hashes, input commitments) without accessing raw data, ensuring outputs derive from agreed-upon logic.
- Privacy: Inputs, weights, and gradients remain encrypted or masked, relying on cryptographic primitives like homomorphic encryption or secure multi-party computation to isolate sensitive data.
- Trade-offs: Larger networks or deeper layers increase computational overhead, while proof size and verification speed depend on the underlying proof system’s efficiency.
Simply explained, zkML lets someone prove that an AI model's predictions or training results are correct without revealing the underlying data or model details. This is achieved through cryptographic proofs that ensure privacy and accuracy, making the process both secure and verifiable.
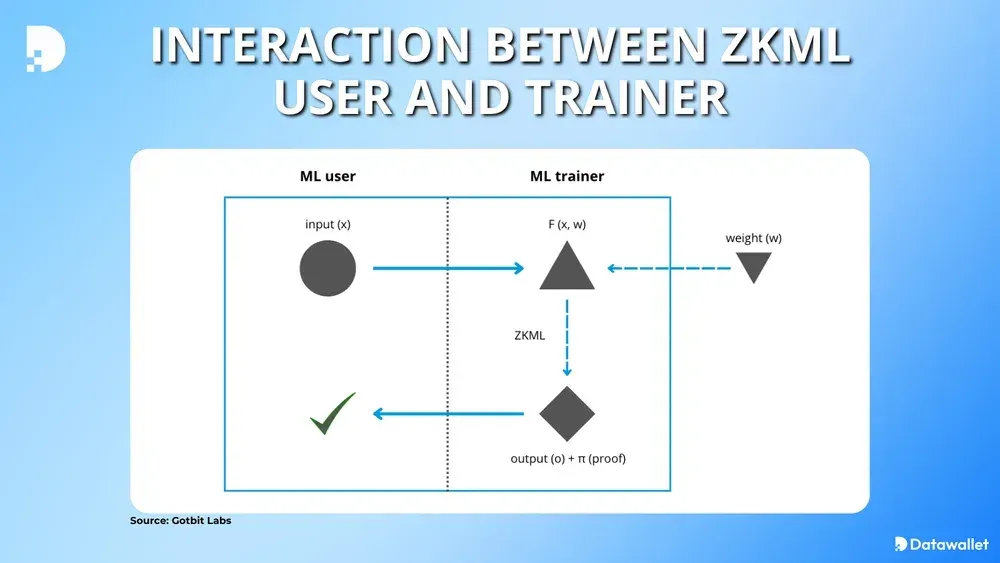
zkML Use Cases
zkML is already proving its utility in crypto, with several projects successfully implementing it or advancing through final testing stages before mainnet deployment.
- On-Chain AI Integrity: Prove trading bots or yield strategies (Modulus Labs’ RockyBot, Giza x Yearn) execute as claimed, preventing hidden manipulation of DeFi markets.
- API Accountability: Expose "black box" ML services by forcing providers to attach proofs showing which models power their outputs.
- Exploit Prevention: Let DAOs programmatically freeze hacked contracts using ZK-anomaly proofs (Aztec Protocol’s research), trained on historical exploit patterns.
- Biometric Upgrades: Users self-update credentials (World's iris codes) via proofs that new biometric templates derive from valid scans, eliminating centralized re-enrollment.
- Medical Confidentiality: Diagnose encrypted MRIs (vCNN) while keeping scans private, replacing compliance paperwork with cryptographic audits.
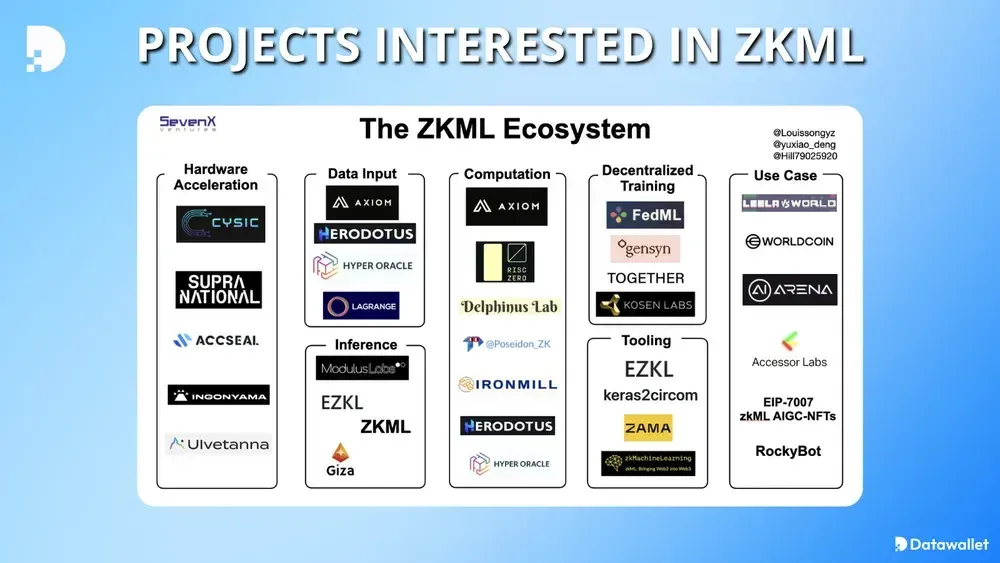
Potential DeFi Implications
When zkML technology matures, it is possible that it will be integrated into even broader use cases across all areas of decentralized finance:
- DeFi Risk Models: Proves correctness of AI-optimized loan collateralization or derivatives pricing (Aave, Synthetix, Hyperliquid, etc.) without exposing proprietary algorithms or user positions.
- DAO Governance: Validates ML-based voting weight calculations or proposal impact forecasts while keeping participant data (e.g., token holdings) private.
- Privacy Coins: Audits transaction anonymity sets (Zcash, Monero) using ML to detect Sybil attacks without compromising user identities or network metadata.
- NFT Valuation: Cryptographically verifies rarity scores or dynamic pricing algorithms (Pudgy Penguins, Azuki NFT) without leaking proprietary valuation logic.
- Cross-Chain Oracles: Secures ML-powered data feeds (Chainlink, Band Protocol) by proving data aggregation integrity across chains without revealing node inputs.
- ZK-Rollups: Enables ML-optimized transaction batching (ZKsync, StarkNet) with proofs ensuring fair ordering/fee calculations without exposing user activity patterns.

Current State of zkML
zkML is 2024’s answer to Silicon Valley’s trust deficit, propelled by crypto giants like Polychain and a16z funneling millions into startups such as Modulus Labs, World (formerly Worldcoin), and Ingonyama to advance on-chain AI privacy and verifiability.
While zkML technology still struggles with ChatGPT-scale models, 2025 could mark a turning point. The US government’s $500 billion Stargate data center initiative, spearheaded by President Trump, and new entrants like China’s open-source AI models from DeepSeek are set to push faster innovations.
Risks and Concerns
zkML’s cryptographic guarantees come with non-trivial risks that could stall enterprise adoption or expose systemic vulnerabilities.
- Exponential Costs: Proving complex models (GPT-4o, etc.) remains prohibitively expensive, with hardware constraints (see Ingonyama’s ZKPU) limiting ROI niche use cases.
- Centralization Risks: GPU/ASIC dependency may consolidate power among a few chipmakers (e.g., Nvidia), contradicting decentralization ideals.
- Security Theater: Poorly implemented circuits, like untested EZKL compilations, risk “verified” outputs that mask data leaks or model flaws.
- Regulatory Ambiguity: Early mandates (mainly EU’s AI Act for now) could force subpar zkML integrations, creating compliance burdens without real security benefits.
- Adoption Friction: Firms like Walmart face talent shortages to operationalize zkML, despite Modulus Labs proving its supply chain value.
Final Thoughts
zkML is a fundamental step in AI accountability but remains constrained by scalability, as current GPU-accelerated proofs struggle to handle trillion-parameter models.
Investors targeting infrastructure options, see zkML as the critical audit layer for industries like DeFi and drug discovery, where AI transparency safeguards billions.
Regulators face a delicate balancing act: excessive oversight risks choking innovation, while inaction could allow unverified AI to undermine trust in vital systems.
.webp)
Written by
Jed Barker
Editor-in-Chief
Jed, a digital asset analyst since 2015, founded Datawallet to simplify crypto and decentralized finance. His background includes research roles in leading publications and a venture firm, reflecting his commitment to making complex financial concepts accessible.